Arman Eshraghi, CEO and Founder of Qrvey, hosts a podcast, “SaaS Scaled.” Our latest episode featured Peter Voss, Founder/ CEO/ Chief Scientist, AGI Innovations & Aigo.ai, developers of the first and only “Chatbot with a Brain.” You can watch or listen to the podcast here and we’ve covered some highlights of their discussion below.
The 3 Waves of AI
The field of artificial intelligence has been around for about 60 years, but there’s a useful way to slice it up into different phases or eras. DARPA called this, “The 3 Waves of AI.”
- Rule-Based Expert Systems
What people were working on for the first 40 years or so is really rule-based, logic-based, expert systems written to solve problems. A good example of that would be IBM’s Deep Blue, which became the world chess champion in 1997.
- Statistical Systems
The second wave really hit us like a tsunami 10 years ago, which is all about big data statistical systems. That’s when companies like Google and Amazon figured out how they could use the massive amounts of data that they’ve accumulated and massive amounts of computing power to build useful AI systems.
Examples of that would be the advances made in autonomous driving, image recognition, language translation, and speech recognition. All of those areas have benefited tremendously by this statistical big data approach. The most recent variation that most people are familiar with is ChatGPT. It’s a variation called generative AI, but it’s still statistical AI using massive amounts of information to build models that can then do useful things.
- Cognitive AI
The third wave that will get us to human-level intelligence is cognitive AI, systems that are inherently geared to the requirements of human intelligence.
Statistical AI systems require hundreds or thousands of examples for them to work, so they can’t easily learn quickly. But cognitive AI works more like a human where it can learn very quickly, and it can learn incrementally and adjust its knowledge. You don’t need massive amounts of information.
And that’s really what we’ve been working on for the last 20 years, but the mainstream of AI, almost all of the work in AI is really on statistical big data approaches.
Achieving hyper-personalization while maintaining confidentiality & privacy
Our hyper-personalization approach is to totally isolate the information that you learn from each individual. The way we do that is, you can think of our brain having three layers.
- The inner layer is information that applies to everyone, every company, every person. That’s just common sense knowledge that you need to have, how to have a conversation, how to greet people.
- The middle layer is the information that’s specific to a company, so that would be their business rules, products, and it may include proprietary information. You want to keep that special for each company that is using it. That has to be trained on the company’s information, and typically there’s also integration to the backend system. So, there’ll be APIs that can also get the latest product information availability, order status, etc.
- The outer layer is unique for every single user that uses the system.
These three layers are integrated in practice as you’re having a conversation, but they are completely isolated so that what one customer tells you isn’t going to be known by any other customer. That is the way we can achieve hyper-personalization and still have a high level of confidentiality and privacy.
Our technology really is totally agnostic in terms of use cases, companies, industries, and so on. It is a very general conversational AI technology.
What are use cases for a chatbot with a brain behind it?
Our technology really is totally agnostic in terms of use cases, companies, industries, and so on. It is a very general conversational AI technology, that can be used by:
- Banks
- Medical applications
- Helping salespeople integrate with Salesforce and manage their process
- Coaching people with diabetes, learning about their food preferences
- A front end to complex software
With complex software, users don’t get to know all of the menu options. If you have a conversational AI like Aigo integrated into your software, you can just tell it what you want to do. As a co-pilot, it can then get you to the right place or actually even do it for you.
Predictions for the future
At the moment, there’s still a fair amount of human labor involved to understand the customer’s business rules, gather all of that information, train the system, and integrate it into their back end. In the future, we see the technology itself becoming more and more capable of understanding requirements. The chatbot technology will interview the customer and gather all the information, such as call center training material, and then configure itself to a large degree so that the implementation will be much quicker and less expensive.
A True Personal assistant
Now, on the individual consumer side, what we’re extremely excited about is the ability to offer a personal assistant, hopefully in the near future. Three different meanings of the word personal come into play here:
- You own it. It serves your purpose, your agenda. It’s not owned by some mega corporation, like Alexa or Siri. I mean, Siri probably won’t tell you about the latest Samsung phone, and Alexa probably won’t tell you about the specials at Walmart, and so on.
- Hyper-personalization. You’re not a demographic. You are an individual and it’ll learn as you use the system. It’ll learn your preferences, history, and likes, who you interact with, and it will do things for you, basically, that you don’t have to struggle with chatbots and websites and things.
Privacy. It will only share things that you tell it to share with whoever. So certain things you share with your spouse, other things you share with your coworkers, and some things you share with Amazon.
How do you diagnose & correct AI errors?
Statistical approaches in generative AI are really black boxes. Even the CEO of OpenAI, Sam Altman, says we don’t know how these things come to what they say, and that’s inherent in those statistical models. They basically just have this huge network that’s inscrutable.
Now with Aigo’s cognitive AI approach, we actually have a scrutable knowledge graph. You can actually trace down everything that the system knows and does. We can trace exactly why it came to a certain conclusion or said a certain thing. It might require some sophisticated tools to analyze it because it could be a very complex decision that it made, but everything is scrutable inherently.
The beauty of that is if it has some incorrect information, such as a procedure or business role that has changed, you can actually go in and specifically change that piece of information. Whereas statistical systems, second wave type systems, have the problem that if you do additional training, they can suffer “catastrophic forgetting.” That’s a problem that’s been known in neural networks for a long time. Things they knew beforehand that you could rely on suddenly change as you train and it’s a whole black box. That’s another reason why this second wave, the statistical or generative AI, is not going to get us to human level AI because it’s not auditable. It’s not explainable and you can’t fix things or track down things.
Use cases to improve human life
Looking further out, our ultimate vision as we get closer to human level intelligence is to have AI researchers. Imagine you train one cognitive AI to be a cancer researcher and then make a million copies. You now have a million PhD level cancer researchers chipping away at the problem. You could take this approach and make progress designing better, more effective batteries, other problems with energy, pollution, or even governance. This will enable us to apply a lot more intelligence to problems facing us, and that can improve human life.
Will we have AI teachers?
There’s currently a big push to use large language models in education. Now, it still has limits. It can be a fantastic tool, but it still suffers the problem of not being hyper-personalized. The other issue is that it can get things wrong, so you need to be careful. It must either have so many guardrails that you’re crippling it, or without guardrails, it might give you misleading information. Future cognitive AI will overcome those limits.
We’re also talking to universities about a hyper-personalized assistant that could help individual students get oriented, find their way around, and help with their studies. To us, it’s a very exciting application and we hope we can get into that sooner rather than later.
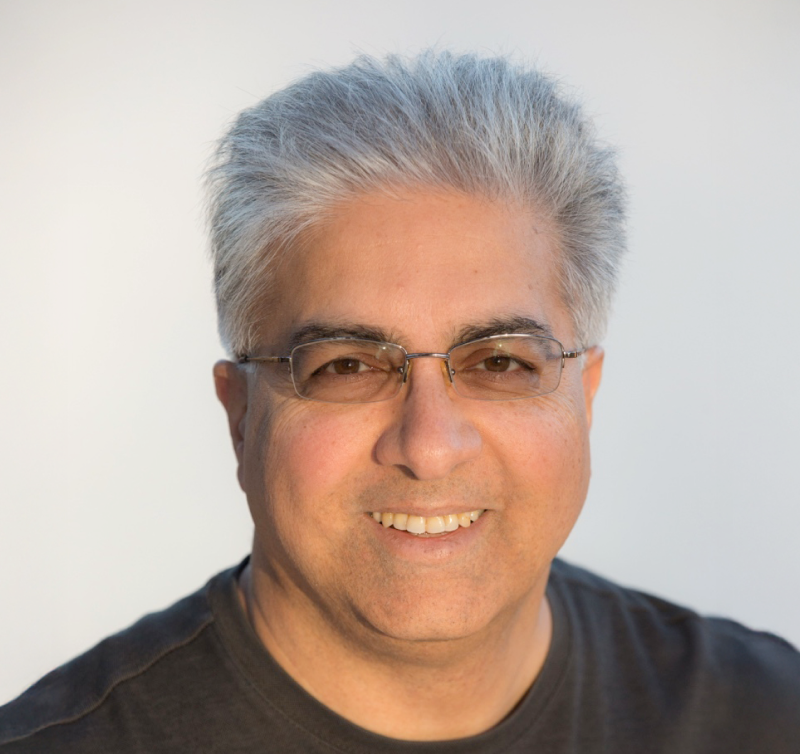
Arman Eshraghi is the CEO and founder of Qrvey, the leading embedded analytics solution for SaaS companies. With over 25 years of experience in data analytics and software development, Arman has a deep passion for empowering businesses to unlock the full potential of their data.
His extensive expertise in data architecture, machine learning, and cloud computing has been instrumental in shaping Qrvey’s innovative approach to embedded analytics. As the driving force behind Qrvey, Arman is committed to revolutionizing the way SaaS companies deliver data-driven experiences to their customers. With a keen understanding of the unique challenges faced by SaaS businesses, he has led the development of a platform that seamlessly integrates advanced analytics capabilities into software applications, enabling companies to provide valuable insights and drive growth.
Popular Posts
Why is Multi-Tenant Analytics So Hard?
BLOG
Creating performant, secure, and scalable multi-tenant analytics requires overcoming steep engineering challenges that stretch the limits of...
How We Define Embedded Analytics
BLOG
Embedded analytics comes in many forms, but at Qrvey we focus exclusively on embedded analytics for SaaS applications. Discover the differences here...
White Labeling Your Analytics for Success
BLOG
When using third party analytics software you want it to blend in seamlessly to your application. Learn more on how and why this is important for user experience.