Businesses navigate a vast ocean of data today. Analytics software serves as the compass guiding decision-makers toward their destination. However, just as a sailboat and a cargo ship require different navigational tools, business intelligence (BI) and embedded analytics serve distinct purposes.
For software product managers charting their course, understanding the nuances between these two types of analytics is crucial. This knowledge not only helps in avoiding treacherous waters but also ensures a smooth sailing experience for end-users.
Let’s dive into the key differences between embedded analytics vs business intelligence. We’ll explore why this distinction matters for product strategy and user satisfaction.
What is Embedded Analytics?
Embedded analytics is when software teams include analytic features as part of another application.
The Dresner Wisdom of Crowds® 2023 Embedded Business Intelligence Market Study has stats on usage. Current use of embedded BI is at 49 percent and adoption plans remain strong. Additionally, 68 percent of industry respondents say embedded BI is critical or very important.
With embedded analytics, SaaS app end users can have the power of BI right within their app. This is a key component of the Analytics as a Service model, which empowers users to analyze the data they create inside that app.
Switching between apps isn’t too difficult, especially with ‘Windows + tab’. However, having information within the main app is the best user experience.
Embedding insights eliminates friction and eases workflows while enabling users to get more value from your app.
Oracle’s recommendations for a BI solution include looking for a single BI platform. “Many organizations have a legacy business intelligence ecosystem featuring multiple solutions for reporting, discovery, analysis, and other functions. Working with all those solutions can be expensive and require extensive technical knowledge. Multiple solutions can also create compatibility issues.”
External vs. Internal Use
- Internal use cases involve using a BI application within departments or line-of-business groups.
- External use cases involve using embedded analytics with users of a SaaS platform. This means the company that purchases the software is NOT the end user.
Vendors typically sell BI applications to individual companies to help them generate actionable insights for operational purposes. Users from all parts of the business use the BI app to analyze data for business processes.
This is distinct from developers who insert the analytical components into their web applications. When software providers include analytics, protecting data across tenants presents a special challenge not seen in internal use.
When tenants access the same database instance, strict access controls must prevent visibility into other tenants’ data. Maintaining security at scale is paramount.
Unfortunately, for most BI vendors, multi-tenancy is not native. When companies try to embed BI, it causes big problems since that’s not its intended use.
In, “How to Select an Embedded Analytics Product,” author Wayne Eckerson talks about how embedding is not part of the BI software DNA. “Converting a stand-alone, commercial product into one that can be easily embedded in both single- and multi-tenant environments with full fidelity is challenging.”
Embedding a traditional BI in a SaaS application leads to issues that waste time and money. Supporting both viewing and authoring in a multi-tenant environment requires exhaustive development efforts.
Qrvey’s goal is to have you build less software and deliver more value to users.
1. User Security
Regardless of the use case, using a lowest-level access philosophy is key.
Organizations must protect sensitive data and consistently enforce data protection. Industries such as healthcare have strict data regulations such as GDPR and HIPAA. Systems that contain this information should be well-equipped with role-based access control to assure proper security.
SaaS apps must empower administrators with record- and column-level security to restrict data access at granular levels. SaaS vendors must also ensure data security and access control are always up to date. Embedding third-party analytics can be challenging, especially when sending data to a third-party cloud. This can create more difficulties for a SaaS company.
Embedded analytics BI software must support multi-tenant data security. Ideally, it also inherits your security model, including all of your rules and policies.
BI software, on the other hand, is all seat-based licensing. Therefore, users get permission to a dataset at the user profile. This model does not work in a SaaS application with an unknown number of users.
Data security and governance are the main reasons Qrvey deploys analytics to your cloud-native SaaS environment. Because Qrvey is self-hosted, your data never leaves your account. Your analytics can run entirely inside your environment, inheriting your security policies, which is optimal for SaaS applications.
2. Technical Architecture
Many traditional BI products do not use cloud-native architectures given how old they are. Traditional business intelligence software can typically embed dashboards. Some can even embed individual widgets (charts).
For example, many traditional BI tools rely on iframes for their embeds, which raises serious security concerns. This works for intranets like SharePoint, but SaaS teams know it creates buying hurdles.
But perhaps the biggest hurdle is that legacy BI is almost always server-based hosting. It’s incredibly expensive to scale. It’s one of the main reasons Qrvey is container-based with on-demand services.
When you’re going to host yourself, you’ll come across cloud BI vs traditional BI. They have differences, but in the end, scaling has to be a consideration.
3. Performance & Scale
As your user base expands, your app must keep up and scale securely. Embedded analytics should be capable of analyzing hundreds of millions of rows in seconds.
Scalable Data Management, No Data Warehouse Required
A data lake is a central storage for all kinds of data in its original, unstructured form. Unlike traditional data warehouses, data lakes can ingest, store, and process structured, semi-structured, and unstructured data.
A data warehouse is a data store of primarily transformed, curated, and modeled data from upstream systems. Data warehouses use a structured data format. BI tools typically rely on data warehouses as their source for analytical data.
A data lake is better for embedded analytics in multi-tenant SaaS apps for several reasons. If you’re looking for an embedded analytics example that demonstrates how this works in practice, there are numerous real-world scenarios showcasing its advantages.
- Transforming a data lake into a data warehouse requires the work of a data engineer.
- Multi-tenant data lakes simplify scaling applications, whereas data warehouses don’t easily scale out for multi-tenancy without significant development effort.
- Data lakes can handle diverse data formats, including semi-structured and unstructured data. This makes it easier to offer analysis on different data types.
- Data warehouses struggle with row-level security in multi-tenant environments. Every data warehouse solution requires additional efforts to secure tenant-level separation of data. This challenge compounds with user-level access control.
Learn more about Qrvey’s data-as-a-service platform.
Custom Data Models
Many SaaS applications allow users to create custom fields. Many times, those fields are useful for business decisions. However, offering reporting against custom fields in multi-tenant databases is not easy.
This essentially creates custom data models per tenant. Traditional BI companies cannot handle this without a ton of data engineering effort.
Learn more about Qrvey’s custom data analytics solution.
4. Seamless Embedding with Full White Labeling
White-label capabilities enable you to customize the embedded analytics software, so it blends in seamlessly with your application. When you white-label embedded analytics software, you make your charts, reports, and dashboards look like a seamless part of your software. Users are not expecting a third-party plugin after all.
White labeling enables easy branding and customization with your colors, logos, and other elements. The result is a consistent user experience and better usability.
Look for the ability to white-label the following components:
- Logo
- Header style (plays a big role in whether it blends into your app)
- Chart color palettes
- Fonts – type, sizes, color, and weights
- Spacing inside certain charts – helpful when dealing with axis labels with long text
True white-label analytics software opens up the entire user experience to customization. Legacy BI, using iframes, does not offer this level of customization. This is a key buying decision for embedded analytics customers.
In the end…
For SaaS product leaders, choosing a solution focused on embedded analytics solution, like Qrvey, is paramount for several reasons:
- Native multi-tenancy: Qrvey’s architecture supports multi-tenant environments, addressing the unique challenges of SaaS applications. All without the complications that arise from retrofitting traditional BI tools.
- White-labeling: Qrvey offers a fully white-labeled solution for seamless integration, allowing product leaders to provide a better user experience. This enhances user engagement and satisfaction.
- Scalability and performance: With its cloud-native, container-based architecture, Qrvey enables SaaS applications to scale efficiently as user bases grow. This ensures consistent performance without the limitations of server-based hosting.
- Enhanced security: Qrvey’s self-hosted approach keeps data within the SaaS provider’s environment, inheriting existing security policies and simplifying compliance with data protection regulations.
- Flexibility for custom data models: Unlike traditional BI tools, Qrvey can handle the complex, dynamic data models often found in SaaS applications. This includes custom fields for end-users.
- Cost-effective analytics: Reduce the need for extensive data engineering and custom development. Qrvey allows SaaS product leaders to deliver powerful analytics capabilities more efficiently and economically.
In an increasingly competitive SaaS landscape, embedded analytics is no longer just a feature—it’s a strategic differentiator. By choosing a purpose-built solution like Qrvey, product leaders can empower their users with scalable and secure analytics. This helps drive user adoption, satisfaction, and ultimately, business growth.
See how Qrvey can give you a competitive advantage today.
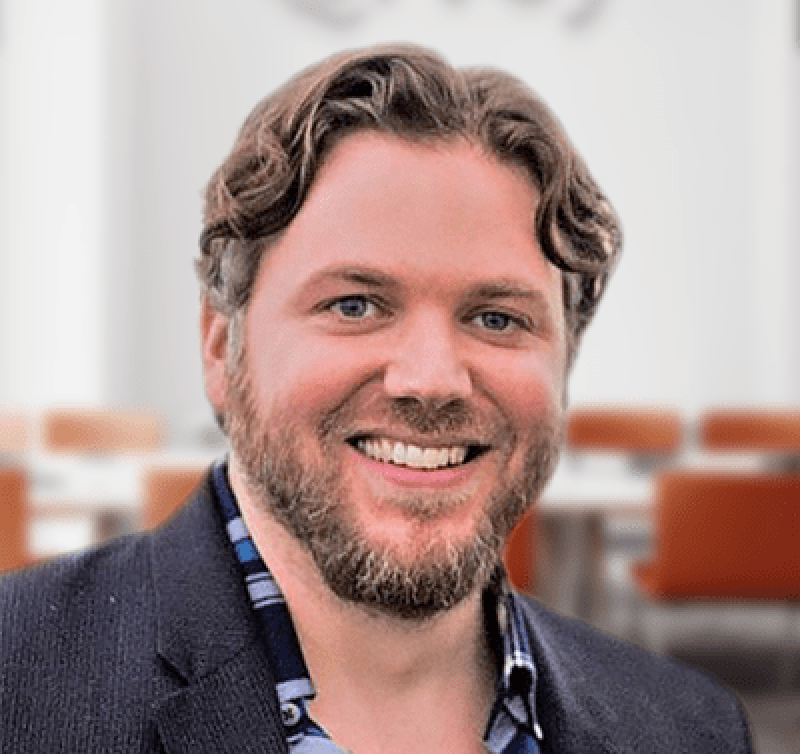
David is the Chief Technology Officer at Qrvey, the leading provider of embedded analytics software for B2B SaaS companies. With extensive experience in software development and a passion for innovation, David plays a pivotal role in helping companies successfully transition from traditional reporting features to highly customizable analytics experiences that delight SaaS end-users.
Drawing from his deep technical expertise and industry insights, David leads Qrvey’s engineering team in developing cutting-edge analytics solutions that empower product teams to seamlessly integrate robust data visualizations and interactive dashboards into their applications. His commitment to staying ahead of the curve ensures that Qrvey’s platform continuously evolves to meet the ever-changing needs of the SaaS industry.
David shares his wealth of knowledge and best practices on topics related to embedded analytics, data visualization, and the technical considerations involved in building data-driven SaaS products.
Popular Posts
Why is Multi-Tenant Analytics So Hard?
BLOG
Creating performant, secure, and scalable multi-tenant analytics requires overcoming steep engineering challenges that stretch the limits of...
How We Define Embedded Analytics
BLOG
Embedded analytics comes in many forms, but at Qrvey we focus exclusively on embedded analytics for SaaS applications. Discover the differences here...
White Labeling Your Analytics for Success
BLOG
When using third party analytics software you want it to blend in seamlessly to your application. Learn more on how and why this is important for user experience.