What to Expect:
- What is a Multi-Tenant Data Lake
- Why is a Multi-Tenant Data Lake Necessary
- How Does a Multi-Tenant Data Lake Relate to Embedded Analytics
- Benefits of Multi-Tenant Data Lakes
- Challenges of Multi-Tenant Data Lakes
- Maximizing Value with Embedded Analytics
- Uniting Multi-Tenancy and Embedded Analytics
- Real-World Use Cases Leveraging Multi-Tenant and Embedded Intelligence
- What are the Requirements of a Multi-Tenant Data Lake for Embedded Analytics
- Architecting Tomorrow’s Data-Driven Applications
Data is powering innovation across every industry. Organizations are racing to build data-driven applications that turn burgeoning information into competitive advantages through actionable insights. However, extracting increasing value from accelerating data volumes poses immense technical challenges.
How can applications scale securely while providing real-time analytics? Multi-tenant data lakes and embedded analytics hold the key.
What is a Multi-Tenant Data Lake
A multi-tenant data lake is a type of data storage that can store information for multiple users or tenants in addition to various data types. Each tenant’s data is kept separate and secure, so they can only access their own information. This allows multiple users or organizations to use the same data while keeping their data private.
In the world of cloud computing and Software-as-a-Service (SaaS) business models, the concept of multi-tenancy is the new normal. The architecture of a multi-tenant data store for analytics is designed to effectively consolidate shared resources, such as storage and computational power.
Why is a Multi-Tenant Data Lake Necessary
This is to efficiently serve multiple customers—each referred to as a tenant. Tenants can be individuals, businesses, or separate organizational units, all sharing the same underlying infrastructure while their data is kept isolated from one another.
Through multi-tenancy, SaaS providers are able to maximize resource utilization, optimize maintenance efforts, and streamline updates which results in significant cost savings. This can also lead to better performance for each tenant as resources are dynamically allocated based on demand.
How Does a Multi-Tenant Data Lake Relate to Embedded Analytics
Moving onto embedded analytics, it refers to the integration of reporting tools, data visualizations, and other analytical capabilities directly within business software applications. This approach is distinct from traditional methods that require users to switch between standalone business intelligence tools and business applications to derive insights from data.
With embedded analytics software, actionable intelligence is brought to users exactly where they need it, within the context of the application they are using. This seamless integration allows users to make data-driven decisions faster and more efficiently.
Embedded analytics is particularly valuable because it democratizes access to data insights. Users without specialized training in data analysis can interpret and utilize complex datasets thanks to intuitive dashboards and reports that are built into the apps they use daily.
When multi-tenant data lakes and embedded analytics come together, the result is powerful: secure, scalable, and performant data solutions that can adapt and grow with increasing customer needs.
For companies utilizing these technologies, it means unlocking integrated intelligence capabilities for all users across the platform without compromising security or performance.
This synergistic pairing is becoming essential in creating a competitive edge and fostering an environment where continuous learning and improvement through data insights is the norm.
Benefits of Multi-Tenant Data Lakes
Multi-tenant data lakes offer compelling advantages but also pose unique design constraints.
Resource Pooling and Elastic Scalability
- Consolidating storage, compute, and administration overhead into shared infrastructure significantly reduces costs for both providers and tenant subscribers as user bases grow.
- Multi-tenant data lakes simplify scaling applications horizontally.
- However, clustered resources must be adequately provisioned to meet concurrency demands across the SaaS tenant base.
Tenant Data Isolation
- While tenants access the same instance, strict access controls prevent visibility into other tenants’ data.
- Maintaining security at scale is critical. Data leak vulnerabilities breaking these barriers carry tremendous risk.
Challenges of Multi-Tenant Data Lakes
Customization Constraints
When traditional business intelligence providers attempt to integrate with multi-tenant architectures, it restricts tenant-specific customizations. This is why most legacy BI solutions struggle to offer embedded analytics to SaaS companies.
Governance Overhead
When SaaS companies attempt to use BI software for multi-tenant reporting, modifying backend data structures impacts all tenant datasets. In fact, it restricts their ability to offer customized data governance and data models per tenant.
Here is where Qrvey stands out. Qrvey offers a multi-tenant data lake that allows for SaaS companies to offer custom data models on a per tenant basis.
Being multi-tenant ready is the primary reason many companies choose Qrvey for embedded analytics.
Maximizing Value with Embedded Analytics
Embedding analytics unlocks significant user experience and adoption advantages:
Contextual Insights Where They Matter
Analytics reach end users directly within regular workflows to guide decisions rather than requiring separate analysis tools.
Data-Driven User Experiences
Integrated analytics provides self-service visibility so users always know where they stand. Management by metrics at all levels fosters a culture of continuous improvement.
Yet, scaling interactive visualizations requires balancing dynamically, freshness, and speed.
Analytics Ownership and Collaborative Decision-Making
Distributed analytics access helps break down information silos and democratizes data insights for all stakeholders. Shared truth establishes alignment.
Uniting Multi-Tenancy and Embedded Analytics
Combining multi-tenant platforms and embedded analytics unlocks scalable and cost-efficient solutions to access insights from data.
Scalable Data Infrastructure
Multi-tenant data lakes provide the foundation for managing massive structured and unstructured data volumes across many tenants while controlling costs.
Air-Tight Security
Strict tenant isolation secures data while robust access controls limit users only to specific permitted data subsets and analytics. Granular permissions prevent leakage across tenant barriers.
Lower Total Cost of Ownership
Shared infrastructure and centralized software instance administration significantly reduce costs for providers.
Customized Insights, Consistent Platform
Each tenant can customize analytics datasets, build dashboards, and tune reports within their space while conforming to shared data models.
Real-World Use Cases Leveraging Multi-Tenant and Embedded Intelligence
Multi-tenant data lake architecture underpins many leading services such as:
- Zendesk serves thousands of accounts with self-service reporting on support interactions leveraging multi-tenancy for scale and security.
- Asana delivers insights to 100,000+ project collaboration customers built within its multi-tenant environment.
- Zuora manages subscription billing analytics across 1000s of platforms.
The combinations of scale, security, and insight accessibility provided by multi-tenant data lakes and embedded analytics solved data challenges holding back application innovation and analytics democratization.
What are the Requirements of a Multi-Tenant Data Lake for Embedded Analytics
A multi-tenant data lake for embedded analytics must meet several key requirements.
- First, it should have robust data isolation capabilities to ensure that each tenant’s data is securely separated from others. This includes implementing strong access controls and encryption mechanisms.
- Secondly, the data lakeshould support efficient and scalable data storage and retrieval. It needs to handle large volumes of data from multiple tenants simultaneously. (Read more on why Qrvey chose Elastic / AWS OpenSearch for it’s multi-tenant data lake)
- Additionally, it should provide flexible data modeling options to accommodate different types of analytics use cases on the tenant level.
- A native semantic layer to map user roles from the SaaS platform to data security controls.
- Lastly, the data lake should offer comprehensive reporting and visualization capabilities, allowing tenants to easily generate and share insights from their data using customizable data visualization tools.
Overall, a multi-tenant data lake for embedded analytics underpins a successful self-service reporting function within a SaaS platform.
Architecting Tomorrow’s Data-Driven Applications
Unlocking value from accelerating data sources is imperative for competitive advantages. Multi-tenant data lake infrastructure with embedded analytics paves the way for custom and scalable data apps serving many tenants with insights needed at their fingertips.
Get started on building or leveraging these technologies by contacting our team for architecture design consulting, troubleshooting current implementations, or technology integration assistance.
The future of data-driven innovation powered by multi-tenancy and embedded insights is here with Qrvey.
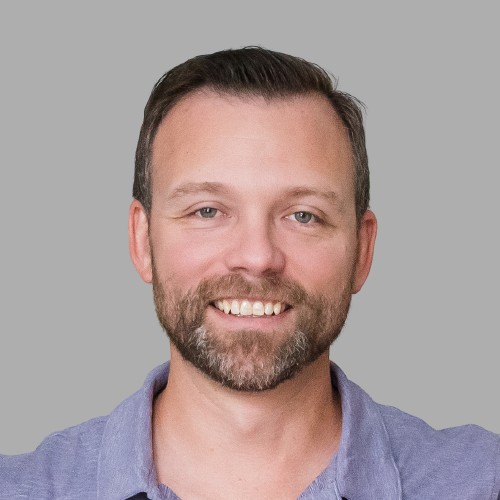
Brian is the Head of Product Marketing at Qrvey, the leading provider of embedded analytics software for B2B SaaS companies. With over a decade of experience in the software industry, Brian has a deep understanding of the challenges and opportunities faced by product managers and developers when it comes to delivering data-driven experiences in SaaS applications. Brian shares his insights and expertise on topics related to embedded analytics, data visualization, and the role of analytics in product development.
Popular Posts
Why is Multi-Tenant Analytics So Hard?
BLOG
Creating performant, secure, and scalable multi-tenant analytics requires overcoming steep engineering challenges that stretch the limits of...
How We Define Embedded Analytics
BLOG
Embedded analytics comes in many forms, but at Qrvey we focus exclusively on embedded analytics for SaaS applications. Discover the differences here...
White Labeling Your Analytics for Success
BLOG
When using third party analytics software you want it to blend in seamlessly to your application. Learn more on how and why this is important for user experience.