Forget hunches and gut feelings. Today’s businesses thrive on a different kind of intuition: data-driven decision-making.
Enter enterprise analytics, the secret weapon that turns mountains of information into clear, actionable insights.
From predicting customer behavior to optimizing marketing campaigns, enterprise analytics unlocks a world of possibilities.
But going from raw data to actionable insights is no small feat. Adding multi-tenant applications can add a layer of complexity that can overwhelm engineering teams.
Let’s explore enterprise analytics in greater detail to see how we can demystify a complex topic.
What is Enterprise Analytics?
Hewlett Packard Enterprise offers the following definition, “Enterprise analytics is the practice of harnessing available internal and relevant external data to inform business decisions and transform data into insight. It is a critical component of any enterprise’s digital transformation efforts.”
Enterprise analytics software enables organizations to realize the value of their data.
In most cases, enterprises have mountains of data. And analytics is the key to gleaning useful information from billions of data points.
Why is Enterprise Data Analytics Important?
There are many ways enterprise analytics is in use today. Perhaps the two most important are here though:
Improved decision-making and efficiencies
With real-time insights and alerts, enterprises can make more data-driven decisions, leading to greater efficiency and productivity. For larger enterprises, small, incremental changes can have huge impacts.
For example, US retailer Target earned $107.41 billion in revenue in 2023. A change that boosts revenue just .05% would equate to over $53 million in additional revenue.
Real-Time Alerts
Timing matters. Threshold triggers are crucial to staying on top of changes. For instance, inventory levels dropping or the output of a machine declining translates to significant expenses.
However, alerts must be immediate to be most valuable. Product reorders need to be prompt to guarantee supply. We must also deal with manufacturing problems immediately to stop a loss of production.
Examples when real-monitoring is useful:
- Monitoring & Optimization: Real-time monitoring of IT infrastructure
- Personalization & Customer Experience: Recommendation engines on ecommerce sites can consider what users are searching and viewing in real-time
- Chatbots: Respond to customer queries with more relevant information
Enterprise Analytics Industry Use Case Examples
Every organization needs information. Whether it’s for soliciting charitable contributions to keeping track of payroll and tax deductions. Some examples are:
Healthcare Analytics
Healthcare data creation continues to grow rapidly. Sources like electronic health records, medical imaging, genomics, health apps, and sensors continue to grow. \
Better reporting has many benefits beyond improving healthcare outcomes, but care providers, and insurance firms as well. Healthcare analytics software can drive enhanced decision-making. This generates predictive insights and prescriptive analytics to improve business efficiency.
When integrating into healthcare SaaS apps, analytics can help detect and prevent fraud and abuse. This happens by analyzing claims data to identify suspicious activities such as false diagnoses, billing issues, or duplicate claims.
Such analyses have the potential to save money for healthcare organizations and protect patients from unnecessary procedures or treatments.
Self-service analytics, and custom reporting capabilities can deliver greater agility and speed to healthcare organizations. Self-service analytics allows users to create reports that show insights for using new information effectively.
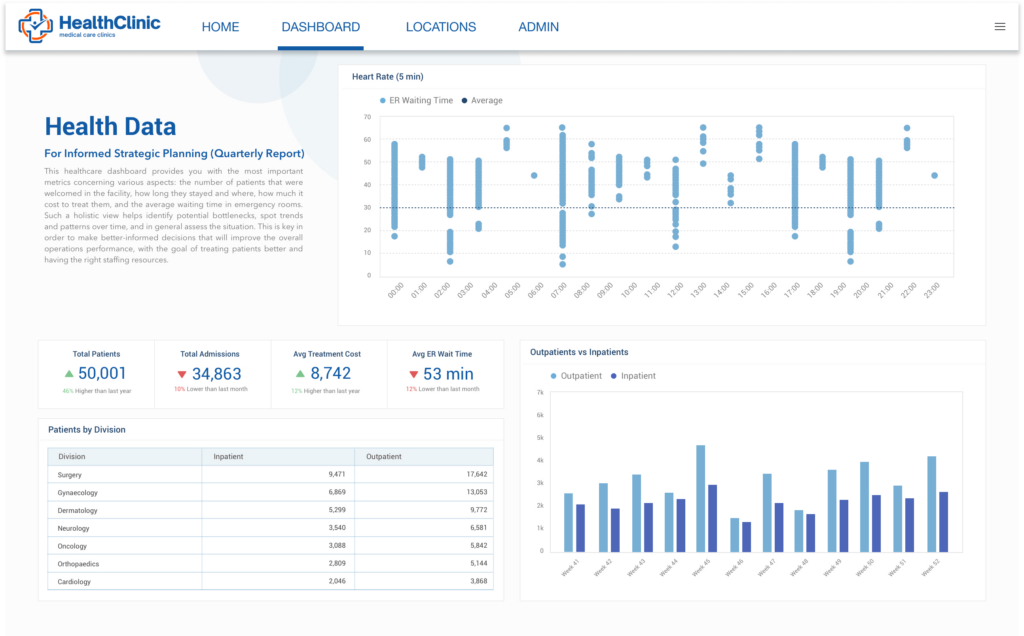
IT and Cybersecurity Analytics
Managing and securing IT infrastructure are difficult challenges for organizations. Infrastructure is becoming more complex, with ever-expanding sprawl. This includes multiple cloud providers, IoT, containers, etc.
Cybersecurity analytics platforms typically generate too many false positives, making it difficult to detect threats and vulnerabilities. And most enterprises are facing these challenges without adequate staff. There are currently over 500,000 unfilled cybersecurity positions in the US alone.
Empowering users with self-service capabilities reduces the burden on IT pros. Analytics can also reduce the burdensome, manual processes that plague cybersecurity teams. Generating security insights faster can enable teams to mitigate the threats that represent the most risk.
Financial Analytics
Financial organizations report paying more than $4 fighting fraud for every $1 of fraud loss. This leaves a substantial opportunity for financial analytics software to uncover potential fraud. AI in particular has great potential to identify patterns and reduce false positives.
Additionally, visualizations of financial data allow for easier comprehension and interpretation of complex data sets. Instead of deciphering numbers and tables, visual representations provide a more intuitive understanding of financial trends and performance.
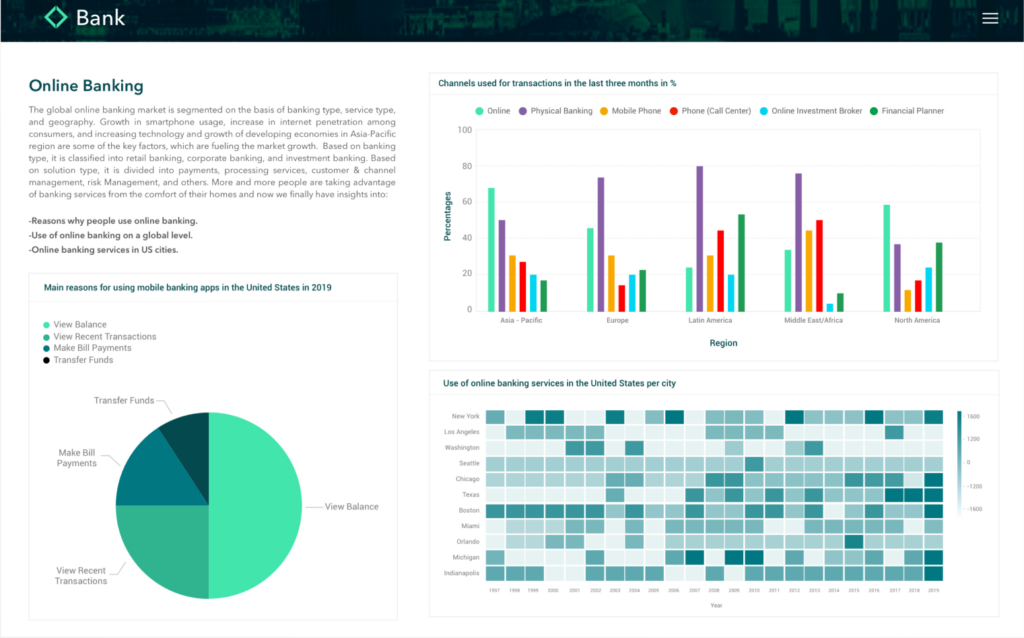
With interactive visualizations, users can manipulate and explore financial data, uncovering hidden insights and patterns that may not be apparent in traditional tabular formats.
Embedded analytics can provide users with immediate access to analytics within familiar systems and accelerate time-to-value.
Read more: Our best embedded analytics tools
Finance professionals often create reports for specific purposes, like audits, taxes, and regulations. An analytics app should let you create reports with precise control over the layout and style of each element. You should be able to adjust each element down to the pixel with pixel-perfect reporting.
Emerging Trends in Enterprise Analytics: Role of AI & Machine Learning
While people know that AI stands for “Artificial Intelligence,” what AI analytics exactly are is less well known. Unfortunately, people often use the term quite loosely. With technology advancing quickly, you need AI components that are strong now and will keep adding value in the future.
Qrvey’s Smart Analyzer unleashes the power of AI to acquire new insights into your data. This feature connects ChatGPT to your chart data, allowing you to ask ChatGPT questions in plain language about your data. Qrvey also connects directly to the AWS AI suite to power real-time machine learning augmentation.
When you embed Qrvey, in many cases, your app automatically inherits those improvements and without any extra work. When AWS updated their NLP service, our app improved without any effort on our part.
Data Security and Governance in Enterprise Analytics
Data governance is complex, composed of various, multi-faceted processes from managing the availability and usability of data, to ensuring ongoing security.
SaaS vendors need to make sure data security and access control is always up to date. Embedding third-party analytics can be challenging, especially when sending data to third party cloud. This can create more difficulties for your organization.
Organizations must protect sensitive data and consistently enforce data protection. Industries such as healthcare have strict data regulations such as GDPR and HIPAA. Systems that contain this information should be well-equipped with role-based access control to assure proper security.
Our take:
Data security and governance are the main reasons Qrvey deploys to your SaaS environment. Because Qrvey is self-hosted, your data never leaves your account. Your analytics can run entirely inside your environment, inheriting your security policies.
This is optimal for SaaS applications. Not only is the application secure, but analytics capabilities are faster to develop, test, and roll out.
With Qrvey’s self-hosted solution, your parent app is in charge of authenticating users. You can simply ‘assert’ securely user identity and their permissions. There’s no need to replicate your existing security schema.
Enterprise Analytics Challenges
Strong analytics provide insights for many users, using different sources for a complete view of all important data, instantly. These attributes each present unique challenges for large enterprises
Finding relevant data
Generally, the larger the enterprise, the larger its data sets. Larger organizations have more types of data too which leads to data quality hurdles.
Expensive line items include:
- Big data storage like data warehouses
- Salaries for the data engineering talent
- The compute power needed to transform the data to a format suitable for analytics.
- And the compute to query large datasets with acceptable latency
Consolidating data sources
In particular, different SaaS products can each generate their own unique data points, from marketing automation to inventory control.
Generating analytics from multiple sources can require building separate pipelines with dedicated ETL for every data source. Data integration can become quite daunting.
Many companies choose Qrvey to consolidate or modernize their legacy systems to solve this problem. Read more about analytics modernization with Qrvey.
Real-time
Real-time data analysis always sounds great and everyone wants it. The reality is that making new data available for reporting right away presents its own set of challenges.
When data needs to fit inside relational data models, there is processing that typically happens first. After completing any transformations, the system sends the data to the analytics database.
The challenge is balancing cost and latency. How “real-time” is actually necessary for the use case? It’s something that needs discussion in the planning phase.
Customing to use cases
When delivering insights for numerous users, enterprise analytics must provide numerous visualizations.
Different users are likely to want different formats, with customizations such as:
- Visualization styles
- Dashboard layouts
- Filters
Self-service capabilities are vital to equip each user with the analytics that meet their unique, changing needs. Those capabilities should also be accessible to non-technical users.
Software with easy, drag-and-drop interfaces see much higher adoption rates. As opposed to empowering only developers and super-admins which limits use cases.
Discover how Qrvey enables Impexium to go to market quickly and get analytics into the hands of customers.
Enterprise Analytics Platform: Features You Need to Have
Businesses must examine data from different systems. They must then create reports and dashboards for employees, partners, and clients. Enterprise analytics software should include functionality such as:
- A variety of data visualization types, such as KPIs, tabular data tables, and heatmaps
- Interactivity such as filtering and drilling down
- Reporting on a broad scope of data sets for full visibility
- Ability to set alerts and notifications, and create workflow automation
- Customizations, such as a personalized dashboard layouts and inclusion of custom fields
- Data write-back capabilities to build workflows
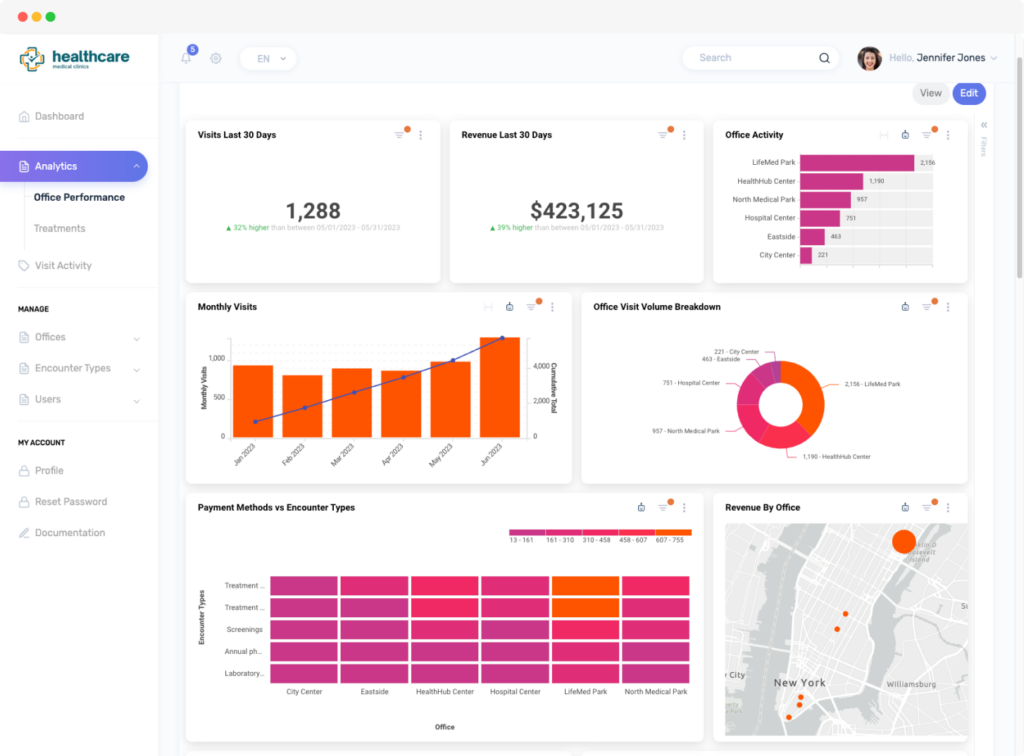
When it comes to the best embedded analytics tools, features to consider include:
- Javascript only, no iframes for security reasons
- UX integration for a seamless experience for your end users
- Pricing and licensing for SaaS providers
- Scalable to billions of records
- Easy multi-tenant configuration, ensuring tenant data security
- Integration to your dev environments, while inheriting your security policies and integrating to your SDLC process
Comparative Analysis
Let’s dive into each of these features and see how Qrvey stacks up against the competition. While there are numerous enterprise analytics solutions available, Qrvey has many attributes that make it the superior choice.
Built Exclusively for Embedded Analytics
Qrvey starts with an exclusive focus on cloud-native embedded analytics. This focus enables Qrvey to meet the needs of SaaS providers – both technical architecture and pricing/ licensing needs.
Fully Embeddable
Full embedding of analytics should include seamless interaction with the different components inside another software or portal.
Many embedded BI tools can embed dashboards and some can embed individual widgets (charts). However, the functionality often fails to meet the needs of SaaS end users.
Also, many traditional BI tools rely on iFrames for their embeds. Most infosec teams struggle to approve iFrame-based solutions due to security concerns. Others that do support JavaScript widgets may lack customization options.
- PowerBI, while always a Microsoft product, wants its customers to use it alongside Azure
- Amazon QuickSight uses iFrames and does not offer full white labeling of dashboards
Just to site a couple examples. If you’re deciding between platforms, it’s worth exploring a detailed comparison like Qrvey vs QuickSight to understand how different embedded analytics solutions stack up.
Enterprise Analytics Pricing and Licensing
Qrvey knows that SaaS vendors are much better off without per-user fees.
SaaS providers aim to increase the usage of their apps. Empowering users to create their own dashboards can make your app useful to more employees within each organization. This also increases satisfaction and the “stickiness” of your application.
It’s reasonable that as your usage of analytics increases, the cost will increase. However, that increase should be proportionate. Unfortunately, with the way some embedded analytics vendors price their products, costs add up too fast to sustain.
For example:
- Power BI charges an additional per-user licensing fee of $15 per month for automation modules
- Qlik has a traditional user-based BI license model with creator and reader licenses available
- Sisense does not offer unlimited user licensing
- Tableau complicates pricing even further. They separate pricing for certain modules and bundles tied to your Salesforce or SQL Server licenses
- Looker requires viewer licenses for anyone who views an embedded dashboard behind a login
Scalability & Performance
Enterprise analytics should empower a broader expanse of use cases, making your app valuable to more people. Your app must keep up with growing demand and scale securely.
Look for a platform that can analyze hundreds of billions of rows in seconds. It should enable use cases such as data augmentation, analysis, and predictive analytics.
Qrvey scales thanks to cutting-edge on-demand services and technology. With Qrvey, your app can keep up with growing demand, scale securely and cost-effectively. Qrvey’s built-in multi-tenant data lake means managing data for multi-tenant analytics gets much easier.
Easy Multi-Tenant Configuration
Most SaaS companies fail with traditional BI software with their lack of multi-tenant security controls.
This makes embedding in a SaaS application a serious challenge, as it requires both additional time and money.
Business leaders often regret enabling both viewing and creating content in a shared environment due to the high development workload. This decision has caused them to reconsider their approach. They are now realizing the challenges and complexities involved in this setup.
As a result, they are facing difficulties in managing and maintaining the system.
How Does Qrvey Support Users with Tech Support, Training, & Community Resources?
At Qrvey, we understand that building a great, embeddable product is just the beginning. Our users need ongoing support including:
- Training to help them understand all the functionality
- Tech support to answer emerging questions and tackle unique challenges
- Professional services to offload development efforts so internal teams can focus on core app functionality
Users and analysts highly praise Qrvey’s support in addition to all the offerings it provides.
But don’t take our word for it.
The Dresner Wisdom of Crowds® Business Intelligence Market Study states, “In 2023, Qrvey remains above the overall sample for most measures with improvements in sales, value, technical support, consulting services, and integrity.
“It is an Overall Leader in both Customer Experience and Vendor Credibility Models and is best in class for sales professionalism, follow up after the sale, all technical support measures, and four out of five consulting measures.”
Start your free POC today and take Qrvey for a test drive in your environment.
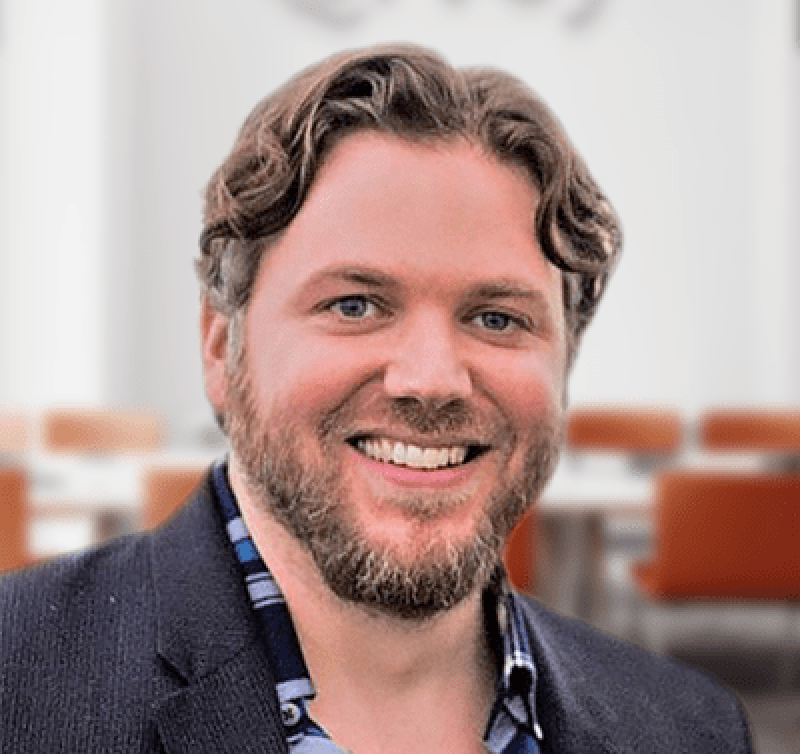
David is the Chief Technology Officer at Qrvey, the leading provider of embedded analytics software for B2B SaaS companies. With extensive experience in software development and a passion for innovation, David plays a pivotal role in helping companies successfully transition from traditional reporting features to highly customizable analytics experiences that delight SaaS end-users.
Drawing from his deep technical expertise and industry insights, David leads Qrvey’s engineering team in developing cutting-edge analytics solutions that empower product teams to seamlessly integrate robust data visualizations and interactive dashboards into their applications. His commitment to staying ahead of the curve ensures that Qrvey’s platform continuously evolves to meet the ever-changing needs of the SaaS industry.
David shares his wealth of knowledge and best practices on topics related to embedded analytics, data visualization, and the technical considerations involved in building data-driven SaaS products.
Popular Posts
Why is Multi-Tenant Analytics So Hard?
BLOG
Creating performant, secure, and scalable multi-tenant analytics requires overcoming steep engineering challenges that stretch the limits of...
How We Define Embedded Analytics
BLOG
Embedded analytics comes in many forms, but at Qrvey we focus exclusively on embedded analytics for SaaS applications. Discover the differences here...
White Labeling Your Analytics for Success
BLOG
When using third party analytics software you want it to blend in seamlessly to your application. Learn more on how and why this is important for user experience.